The fact that neurons in the primary visual cortex respond selectively to the orientation (angle) of contrast edges has been known since the work of Hubel and Wiesel (1962). It was later discovered that these neurons also react to stripe patterns and that their response depends on the distance between the stripes and thus on the “spatial frequency” (the reciprocal of the period length).
22 years ago I wrote my diploma thesis on this topic in the neurophysics group of Prof. Reinhard Eckhorn at the department of physics at Philipps-University Marburg. Among other things, I developed a quick test to determine the spatial frequency selectivity of electrophysiologically derived neuron groups.
The knowledge about the processing of spatial frequencies in the visual system is now also used in artificial neural networks for visual object recognition. An interesting example is a recent paper by Lev Kiar Avberšek, Astrid Zeman and Hans Op de Beeck (2021): “Training for object recognition with increasing spatial frequency: A comparison of deep learning with human vision”. They trained a deep convolutional neural network (CNN) with images with different spatial frequency components. They added low-pass filtered versions of the original images to their dataset and used those in addition to the originals for training their network. Those low pass filtered images contained low spatial frequencies and therefore appeared blurred. They were able to show that with this type of training, the recognition rate for low-pass filtered images from the ImageNet dataset increased from 0 percent to 32 percent.
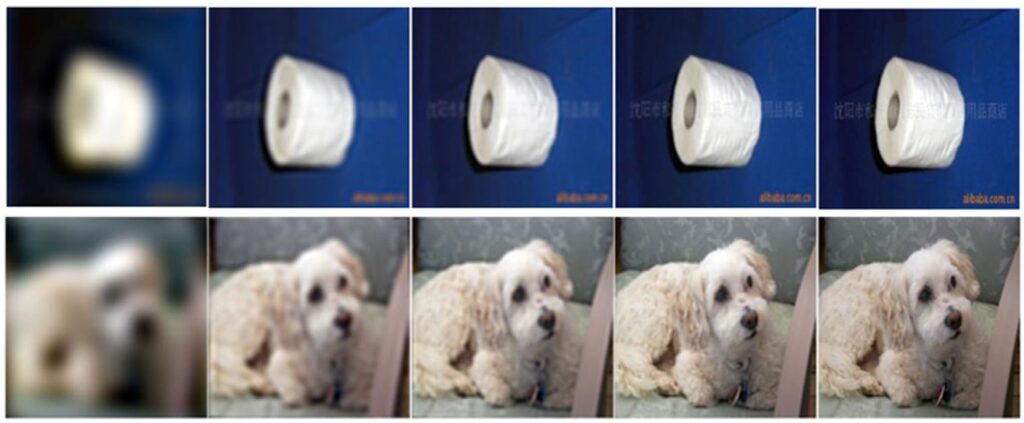